Deep Learning: Creating Machines Of The Future
Deep learning is not actually a technological breakthrough all by itself, it is a branch of learning technology. To understand what it means, one has to first grab the relationship between it, machine learning and artificial intelligence.
What is Machine Learning?
This is a learning technology which permeates major aspect of the modern society. Its use include recognition of objects in images, transcribing of speeches, and production of relevant results of searches, among others. Machine learning technology does these activities by using algorithms to generate insights from data. However, Machine learning is just a set under Artificial Learning.
What then is Artificial Learning?
Artificial learning is one of the biggest breakthrough when it comes to technology. It attempts at making machine to think intelligently and predict more accurately.
DEEP LEARNING AS A BRANCH OF MACHINE LEARNING
Deep learning is not in by itself as said earlier when it comes to Machine learning and Artificial Intelligence (AI).
Having established this clarification, what then is Deep Learning? We mentioned earlier that Machine learning uses algorithms to generate insights from data. Well, Deep Learning specifically uses one of those algorithms called Neural Networks to generate insights. It is one of those algorithms which has been described as being quick to predict things. Machine learning has been for a long time but it had not been able to help achieve task successfully.
You have been wondering why Facebook has been successful in identifying faces in photographs. Well, that is what Deep Learning does. Google also employs Deep learning in managing energy use at its data centers. As a result, Google has been able to reduce energy use. Also, Deep learning makes work accessible.
Before we go on to talk about the things you must know about deep learning, we need to look into what Neural Network mean? Inter-connected artificial neurons form Neural Networks. These neurons pass data among themselves and through weigh and biases, an output is given based on prediction.
NEURAL NETWORKS
Neural nets are algorithms which depend on the mathematical experimentation of Calculus and Algebra. However, most of what occurs is biological representation. It is important to state that neural networks has an inspiration from cerebral cortex while we have layers of interconnected perceptrons at the rudimentary level. To produce successful prediction, input passes through these multi-layers of perceptrons. The output may be a node if the output is just a number while it may be more if the problem is multi-classified. Each of the node has a weight by which it multiplies its input value.
It is necessary to state that the neurons of neural networks have tow linear components labelled as weight and bias. Every input which enters the neuron has a weight attached to it and it represents how genuine or not the input is in relation to its task. The bias on the other hand, is added to the result of the weight multiplication.
LAYERS
Here, we have the input, hidden and output layers as the layers of a Neural Networks. An input layer is the first and it receives the data while the output layer is the final which produces the generated result. In-between these layers is the hidden layer which is otherwise referred to as the Processing Layer. Its function is to perform certain tasks on the incoming data and then pass over to the next layer.
STEPS OF DEEP LEARNING
It is also important to know that deep learning has two steps. The first is analysis of data to generate the algorithm that match the features of that object while the second step is to use the algorithm to identify the object based on real data provided.
DEEP LEARNING ARCHITECTURE
Deep learning architecture has a quite complex categorization. However, it is important that they are understood in order to get to know how its data analysis work. We have:
-Generative Deep Architectures: are intended to attribute the high-level correlation properties of the identified data for pattern analysis, the correlated numerical data and classes. However, through rules like Bayes, it could be turned to a Discriminative Architecture.
-Discriminative Deep Architectures: are meant to provide discriminative power directly for pattern distribution. It features posterior distribution of classes in relation to physical data.
-Hybrid Deep Architectures: While the aim is discriminative power, it is largely aided with the outputs of generative deep architectures as it turned out that the discriminative power is used to study parameters in any of generative architectures model.
6. MACHINE AND DEEP LEARNING SYSTEM
The machine and deep learning system comprises:
-Target function: which is the task being learnt in order to carry it out.
-Performance Element: the components that execute an action in the world.
-Training Data: is the data required to carry out the target function.
-Learning Algorithm: is the algorithm which employs the training data in carrying out the approximation of the target function.
-Hypothesis Space: is the area learning algorithm can consider for likely target function.
7. DEEP LEARNING MODELS
-Deep Feed Forward Networks
Also referred to as Feed-Forward Neural Networks or Multi-layers Perceptrons, they are the most important deep learning models. Its basic goal is to approximate some functions (f). From a clearer perspective, these networks can be described as having input, hidden and output nodes. The approximation is derived when data enters through the input nodes, the hidden nodes processes the data while result is produced through the output nodes.
The models is called feed forward because there is no connection passage through the output is fed back into the network.
-Convolution Networks (CNN/ COV NETS)
This is an artificial feed-forward networks in which the pattern of connection between the neurons is informed by the pattern of animal visual cortex. Each of the cortical neuron reacts to stimuli in their individual receptive field and can be mathematically approximated by the operation of convolution.
The convolution networks is basically patterned to reduce the workload of preprocessing. The four major components of Convolution networks are: Convolutional Layer, Activation Function, Pooling Layer and Fully Connected Layer.
The first Convolution Networks which has helped to promote Deep Learning is LeNets which was employed mainly in character recognition functions.
-Recurrent Neural Networks (RNN)
When output is dependent on the previous computation, Recurrent Neural Networks is needed to perform the task. It has a dependable memory which helps to recover what has been calculated so far. RNN is an architecture which has loops which enable it to read input while also carrying information across neurons.
Recurrent Neural Networks has been useful in machine translations, analysis of video, computer vision, image generation and captioning, among others. One of the good things about RNN is that it allows any number of output and input to be fixed together.
Deep learning models are highly capable of simulating the human brain and can therefore be used to carry out many tasks.
What are the areas of applications?
Since its emergence, deep learning has permeated various fields of human endeavor. The reason is not so far from the fact that it is capable of simulating human brain. Also, it has been found to produce result far better than when carried out by human. Some of the areas where it has successfully produced amazing results are:
-Colour effects on black and white images which uses mostly large convolutional neural networks. Through this, deep learning has been able to recreate images with amazing colour effects.
-Object Identification and Captioning: Using Convolutional Neural Networks, images and objects within photographs have been successfully identified and captioned.
-Automatic Handwriting Generation: With a handwriting example given, it has been able to develop new handwritings for a given word or phrase.
-Automatic Game Playing: With this task, a model learns to play computer games based on the pixels displayed on the screen. This task is quite difficult and it is one of the breakthrough that it is renowned for achieving.
-Generative Model Chatbots: With a training on a serious and real conversational pattern, deep learning has been able to develop a model chatbots that can generate its own answers. The generative bots have been described as the smartest.
Other areas of applications include: Bioinformatics, Audio and Video Recognition, among others. One of the area in which it is yet to achieve this goal is the field of Hand-crafted feature engineering although it has made it less complicated.
Meanwhile, quite a lot are being asked as to the confidence one must put in machine learning technologies. You may as well be worried especially in the strength and durability of machine learning. While some others worry about the displacement of man from his duty. The truth however, is that irrespective of the breakthroughs in machine and deep learning, there is still the unalienable role man has to play. There is still a need for human touch. These machines as at now, cannot compete with the human brain. At most points while carrying out tasks, they still require the touch of human in order to carry out the tasks effectively. Maybe in decades to come, machines would get there. But it is certainly not now.
Machine learning is becoming more and more relevant in the human world. Every day, new architectures are being modelled. Deep learning which is a branch of machine learning is breaking grounds as it is capable of learning and predicting more successfully, from structured and unstructured data.
With the information provided about 7 things you need to know about deep learning, you will find machine learning as a help in respect to multi-tasking and not a threat.
To listen to the audio version of this article click on the play image.
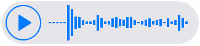
Brought to you by @tts. If you find it useful please consider upvoting this reply.